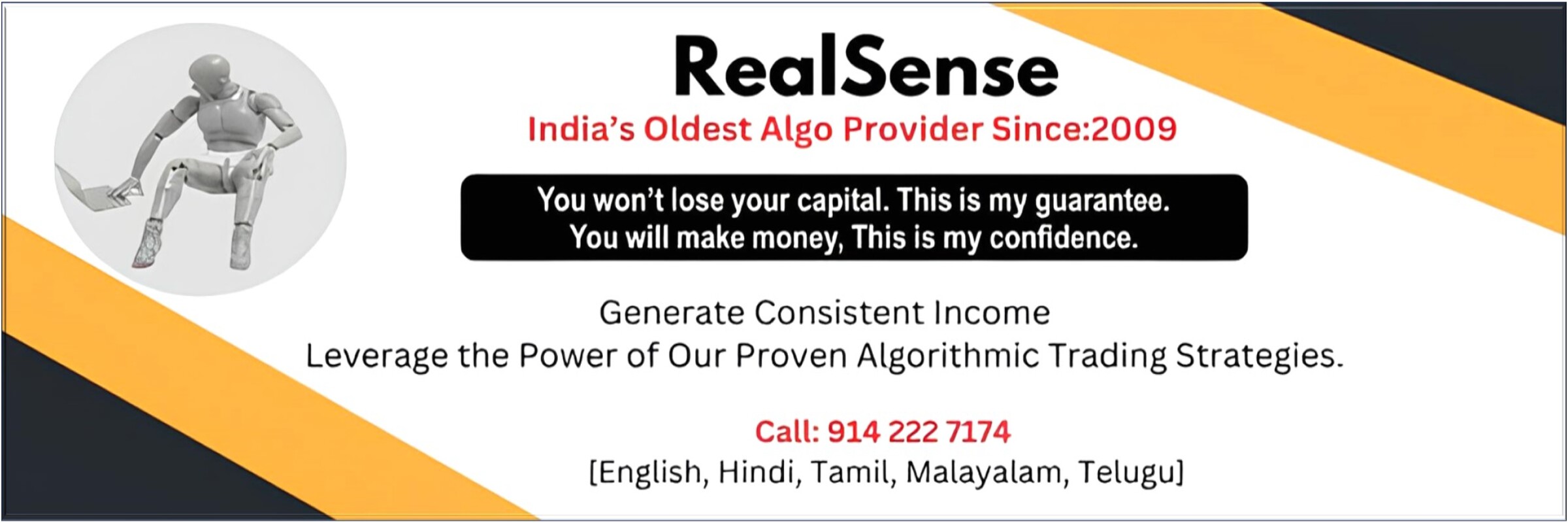
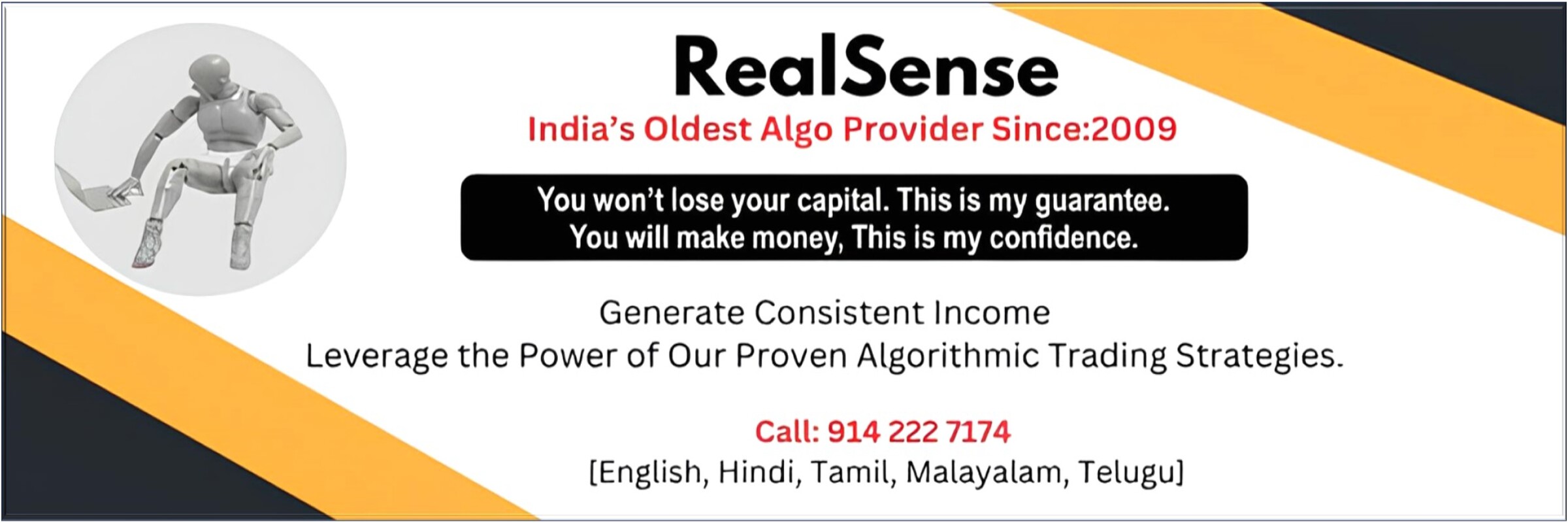
Robot Trading 101: Friend or Foe?
Robot trading (algorithmic trading) automates your investments. But is it right for you? This guide dives into the pros and cons, helps you choose a platform, and unveils common mistakes to avoid. Learn if robot trading can boost your portfolio and get beginner-friendly tips to get started.
Understanding Robot Trading
What is Robot Trading (Algorithmic Trading)?
Robot trading, also known as algorithmic trading, automates investment decisions based on pre-programmed rules. These rules, or algorithms, analyze market data (including price movements, technical indicators, and news events) to generate buy and sell signals. Unlike traditional trading where you manually place orders, robot trading executes trades automatically, 24/7. This allows for faster reaction times to market changes and removes human emotions from the decision-making process.
How Does Robot Trading Work?
Here’s a simplified breakdown of how robot trading works:
- Strategy Development: You define the trading rules using an algorithm. This might involve setting parameters for entry and exit points based on technical indicators like moving averages or relative strength index (RSI).
- Market Data Analysis: The robot software continuously monitors market data feeds, pulling in real-time information about prices, volumes, and other relevant factors.
- Signal Generation: Based on the pre-defined rules, the algorithm analyzes the market data and generates buy or sell signals when specific conditions are met.
- Order Execution: Once a signal is generated, the robot automatically transmits the order to the brokerage platform for execution, completing the trade.
There are various types of robot trading strategies, each suited for different market conditions and investment goals. Some common strategies include:
- Trend Following: Capitalizes on price movements by buying assets in uptrends and selling in downtrends.
- Mean Reversion: Exploits temporary price deviations from the average by buying undervalued assets and selling overvalued ones.
- Arbitrage: Takes advantage of price discrepancies between different markets to generate quick profits.
Benefits of Robot Trading
Robot trading offers several advantages for investors, including:
- Reduced Emotional Trading: By removing emotions from the equation, robots can stick to the predefined strategy and avoid impulse decisions based on fear or greed.
- Backtesting and Optimization: Robot trading allows for historical data analysis (backtesting) to refine and optimize your trading strategy before deploying real capital.
- 24/7 Market Monitoring: Robots can continuously monitor markets for trading opportunities, even outside regular trading hours.
- Faster Execution: Automated trades can be executed much faster than humanly possible, potentially capturing fleeting market opportunities.
- Discipline and Consistency: Robots follow the trading plan strictly, ensuring consistent execution according to the defined strategy.
However, it’s crucial to remember that robot trading is not a magic bullet. Carefully consider your risk tolerance and investment goals before diving in.
Is Robot Trading Right for You?
Pros of Robot Trading
Robot trading offers several advantages, but it’s not a one-size-fits-all solution. Here are some key benefits to consider:
- Reduced Emotional Trading: Human emotions can cloud judgment and lead to impulsive decisions. Robots eliminate these emotions, sticking to your predefined strategy and avoiding costly mistakes based on fear or greed.
- Disciplined and Consistent Execution: Robots follow your trading plan with unwavering discipline, ensuring consistent execution according to your defined parameters. This removes human biases and ensures you don’t deviate from your long-term goals.
- 24/7 Market Monitoring and Opportunity Capture: Unlike humans, robots can tirelessly monitor markets around the clock, identifying and capitalizing on trading opportunities that might arise outside regular trading hours. This can be particularly beneficial in fast-moving markets.
- Backtesting and Strategy Optimization: Robot trading allows you to backtest your strategy using historical data. This enables you to refine your approach, identify potential weaknesses, and optimize your algorithm before risking real capital.
Cons of Robot Trading
While robot trading offers advantages, it also comes with limitations to consider:
- Limited Adaptability to Unforeseen Events: Markets can be unpredictable, and unforeseen events (like economic crises or black swan events) can disrupt even the most well-defined strategies. Robots may struggle to adapt to such situations, potentially leading to losses.
- Technological Reliance and Potential for Errors: Robot trading software relies heavily on technology. Technical malfunctions, bugs, or data feed disruptions can lead to unintended consequences and impact your trades.
- Potential for Overfitting: Overfitting occurs when a strategy is optimized to historical data and performs poorly in real-time markets. Backtesting is crucial to avoid this, but it doesn’t guarantee future success.
- Requires Ongoing Monitoring and Maintenance: Robots aren’t “set it and forget it” tools. Markets and trading conditions evolve, so you’ll need to monitor your robot’s performance and potentially adjust the strategy over time.
Consider Your Investment Style
Before diving into robot trading, it’s essential to assess your investment style. Here are two key factors to consider:
- Risk Tolerance: Robot trading can involve inherent risks, just like any investment strategy. Evaluate your risk tolerance and choose a robot strategy aligned with your comfort level for potential losses.
- Investment Goals: Are you aiming for short-term gains, long-term wealth accumulation, or a combination of both? Different robot trading strategies cater to varying investment goals. Choose one that aligns with your overall financial objectives.
By carefully considering the pros, cons, and your unique investment profile, you can make an informed decision about whether robot trading is the right fit for your financial journey.
Choosing a Robot Trading Platform
Selecting the right robot trading platform is crucial for your automated investing success. Here’s what to consider:
Key Features to Look For
- Strategy Development and Backtesting Tools: A robust platform should allow you to easily build and customize your trading strategies using a visual interface or coding options. Backtesting capabilities with historical data are essential to evaluate your strategy’s performance before deploying real capital.
- Asset Selection and Market Connectivity: Ensure the platform supports the asset classes you’re interested in trading, such as stocks, forex, or cryptocurrencies. Additionally, verify seamless integration with your preferred brokerage account for smooth order execution.
- Risk Management and Order Controls: Look for features like stop-loss and take-profit orders to automatically limit potential losses and lock in gains. Advanced options might include trailing stop-loss for dynamic protection and position sizing tools to manage risk based on account balance.
Security and Regulation
Security is paramount when trusting a platform with your investment decisions. Here’s what to assess:
- Data Encryption and User Authentication: The platform should utilize robust data encryption protocols to safeguard your financial information and login credentials. Multi-factor authentication adds an extra layer of security to prevent unauthorized access.
- Regulatory Compliance: Verify that the platform operates under the regulations of a reputable financial authority. This ensures adherence to industry best practices and consumer protections.
Fees and Costs
Robot trading platforms typically have various fee structures. Here’s a breakdown:
- Subscription Fees: Some platforms charge monthly or annual subscription fees for access to their features and functionality.
- Transaction Fees: These fees are charged per trade executed by the robot. They might be a fixed amount or a percentage of the trade value.
- Brokerage Commissions: Remember that your chosen brokerage might also have its own commission structure for trade execution, independent of the robot platform fees.
Carefully compare the features, security measures, and fee structures of different platforms before making your decision. Opt for a platform that aligns with your needs, budget, and risk tolerance.
Common Robot Trading Mistakes (and How to Avoid Them)
Even with automation, there’s room for error in robot trading. Here are some common mistakes to steer clear of:
Setting Unrealistic Expectations
Robot trading isn’t a magic bullet to guaranteed riches. Entering with unrealistic expectations can lead to disappointment and potentially risky behavior.
- Understand Market Volatility: Markets are inherently volatile, and even the best strategies will experience periods of losses. Focus on long-term performance and accept that setbacks are part of the game.
- Set Realistic Goals: Define achievable goals aligned with your risk tolerance and investment horizon. Don’t chase unrealistic returns that could lead to overly aggressive strategies.
Not Backtesting Your Strategy (Simulating Past Performance)
Backtesting is the process of simulating your robot’s trading strategy using historical market data. This allows you to evaluate its performance before risking real capital.
- Identify Strengths and Weaknesses: Backtesting helps you identify periods where your strategy might have performed poorly. Use this information to refine your approach and address potential shortcomings.
- Avoid Overfitting: Overfitting occurs when a strategy performs well in backtesting based on historical data but struggles in real-time markets. Backtesting with diverse datasets can help mitigate this risk.
Ignoring Risk Management
Risk management is crucial in any investment strategy, and robot trading is no exception.
- Stop-Loss and Take-Profit Orders: Implement stop-loss orders to automatically exit positions when they reach a predefined loss threshold. Take-profit orders lock in gains when prices reach your target level.
- Position Sizing: Allocate a calculated percentage of your portfolio to each trade, avoiding overexposure to any single position. This helps manage risk and potential losses.
- Regular Monitoring and Maintenance: Markets and trading conditions evolve. Regularly monitor your robot’s performance and be prepared to adjust the strategy or parameters as needed.
By avoiding these common pitfalls and implementing sound risk management practices, you can increase your chances of success in the world of robot trading.
Getting Started with Robot Trading (For Beginners)
The world of robot trading can be exciting, but it’s crucial to approach it with a measured and educational mindset. Here are some steps to guide you as a beginner:
Develop a Basic Trading Strategy
Before diving into code or platforms, take a step back and define your trading strategy.
- Identify Your Investment Goals: Are you aiming for short-term gains, long-term wealth accumulation, or a balance of both? This will influence your strategy selection.
- Research Different Trading Styles: Explore various robot trading strategies like trend following, mean reversion, or arbitrage. Understand the underlying principles and how they align with your goals and risk tolerance.
- Keep it Simple: As a beginner, it’s wise to start with a simpler strategy. Complex algorithms can be more prone to errors and require advanced knowledge.
Paper Trading Before Going Live (Simulate Trading with Virtual Funds)
Paper trading allows you to test your robot’s strategy using a simulated trading environment with virtual funds. This is a fantastic way to gain experience and confidence before risking real capital.
- Practice Risk Management: Paper trading is a safe space to experiment with stop-loss orders, position sizing techniques, and backtesting your strategy in a risk-free environment.
- Develop Discipline and Emotional Control: Paper trading allows you to practice making disciplined trading decisions based on your strategy, avoiding impulsive reactions based on emotions.
- Evaluate Platform Functionality: If you’re using a robot trading platform, paper trading lets you explore its features, interface, and order execution process before investing real money.
Start Small and Scale Gradually
Once you’re comfortable with paper trading and have refined your strategy, it’s time to take the leap to live trading. However, a cautious approach is essential.
- Start with a Small Investment: Don’t risk a significant portion of your capital right away. Begin with a small allocation to test your robot’s performance in real-time markets.
- Monitor Performance Closely: Continuously monitor your robot’s trades and adjust the strategy or parameters as needed. Remember, markets are dynamic, and what works in paper trading might require tweaks in live environments.
- Scale Up Gradually: If your robot performs well with a small investment, you can gradually increase the allocated capital over time. This measured approach allows you to manage risk and build confidence in your automated trading journey.
By following these steps and prioritizing education over quick wins, you can increase your chances of success in the world of robot trading. Remember, this is a marathon, not a sprint. Be patient, learn from your experiences, and continuously refine your approach.